12 Απριλίου 2024
Αίθουσα ΕΑ1 στο παράρτημα του Τμήματος Πληροφορικής στην Εθνικής Αντιστάσεως 16
18:00-19:00
Abstract
Η χρήση μεθόδων Μηχανικής Μάθησης (ΜΜ) για την επιτάχυνση της επιστημονικής ανακάλυψης είναι πλέον ευρέως διαδεδομένη, δημιουργώντας νέες προοπτικές σε σχεδόν κάθε επιστημονικό τομέα. Αλλά μαζί με τις νέες προοπτικές έρχονται και καινούριες προκλήσεις όσον αφορά την εκπαίδευση, την αξιολόγηση, την υιοθέτηση και -τελικά- την εξαγωγή επιστημονικών γνώσεων από μοντέλα ΜΜ. Στην ομιλία αυτή θα εξετάσουμε μερικές από τις βασικές πτυχές που πρέπει να ληφθούν υπόψη κατά την εφαρμογή μεθόδων ΜΜ σε επιστημονικές εφαρμογές. Θα συζητήσουμε επιλεγμένα θέματα που σχετίζονται με την αξιολόγηση αλγορίθμων και μοντέλων ΜΜ (model & algorithm evaluation), την εκτίμηση της αβεβαιότητας των προβλέψεων τους (model uncertainty quantification), την ενσωμάτωση επιστημονικής γνώσης στα μοντέλα (encoding of domain knowledge into the models), την ερμηνευσιμότητα των μοντέλων (model interpretability/explainability), την εξαγωγή σχέσεων αιτιότητας (causal inference) και άλλα. Θα συνδυάσουμε τις έννοιες αυτές με παραδείγματα από επιστημονικές εφαρμογές, κυρίως στην Βιοϊατρική και την Αστρονομία.
Short CV
Dr. N. Nikolaou is a Lecturer (Assistant Professor) at UCL’s Centre for Data Intensive Science & Industry (2022- present). His research interests span both the theory of Machine Learning (with a focus on explainable ML, uncertainty quantification, causal inference & resource-efficient ML) and scientific applications of ML (primarily in astronomy, biology, medicine & clean energy). Before his current post, he worked in AstraZeneca Oncology Biometrics R&D as a Senior Data Scientist (2021-22). Prior to this he held a Senior Research Fellow post in the Department of Physics & Astronomy at UCL (2018-21), applying ML methods, with an emphasis on explainable deep learning, to exoplanet detection & atmosphere characterization and before that he held an EPSRC Doctoral Prize Fellowship in the Department of Computer Science of the University of Manchester (2016-18), investigating theoretical aspects of ML (classifier calibration, information theory & model selection). He holds a PhD in Computer Science (Machine Learning) from the University of Manchester (2016). His work has received several awards & distinctions: a nomination for the British Computing Society’s (BCS) 2017 ‘Distinguished Dissertation Award’, the 2017 ‘Steve Furber Medal’ & the 2016 ‘Carol Goble Medal’ by the University of Manchester, a 2016 ‘Best of Computing, Notable Article’ by the Association of Computing Machinery (ACM), a plenary presentation in the 2016 European Conference on Machine Learning (ECML), the Best Poster Award in the 2015 INIT/AERFAI Machine Learning Summer School.
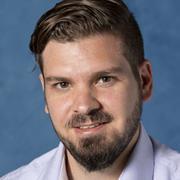